Abstract
The rapid emergence of Bitcoin and other cryptocurrencies has taken many in the energy sector by surprise. This report summarizes complexities and pitfalls in analyzing the electricity demand of new information technology, focusing on Bitcoin, the mostly widely used cryptocurrency. It also gives best practices for analyses in this space, and reviews recent estimates in light of those best practices. Things change rapidly for cryptocurrency, so special care (such as including an exact date for each estimate) is needed in describing the results of such analyses.
The most reliable estimates of Bitcoin electricity use for June 30, 2018 total about 0.2% of global electricity consumption. Because of the collapse in Bitcoin prices in the latter half of 2018, some estimates indicate that this total has begun to decline, though nobody knows if that trend will continue. Future studies of cryptocurrency electricity use can avoid the pitfalls identified in this report by following some simple rules, which the report describes in more detail.
Article Citation
Jonathan Koomey, "Estimating Bitcoin Electricity Use: A Beginner’s Guide 1.0," May 2019.
Table of Contents
Acknowledgements
This report was funded by a grant from Coin Center. Dozens of people asked me to write a report on this topic, but Coin Center was the first to bring funding with its request, and I am grateful for its support. This report was reviewed by a distinguished group of industry experts, including Marc Bevand, Nic Carter (Castle Island Ventures), Patrick Flynn (SalesForce), William Layden (Cube Hydro Partners, LLC), Amory Lovins (Rocky Mountain Institute), Jens Malmodin (Ericsson), Eric Masanet (Northwestern University), John Mercurio and Mikhail Ishmanov (BitFury Group), Evan Mills (formerly at Lawrence Berkeley National Laboratory, now retired), John Pfeffer, Donna Sadowy (AMD), Dale Sartor and Arman Shehabi (Lawrence Berkeley National Laboratory), Harald Vranken (Open University and Radboud University), Bill Weihl (formerly at Facebook and Google), and Henry Wong (formerly at Intel). I’m thankful for the input of these reviewers, but any remaining mistakes are my responsibility alone.
Introduction
The rapid emergence of Bitcoin and other cryptocurrencies has taken many in the energy sector by surprise. Interest in cryptocurrencies exploded towards the end of 2017, leading to questions about how much electricity demand would be affected by this technology. Because energy infrastructure is expensive and hard to build, questions about rapid growth of electricity demand take on particular urgency for utilities and policy makers responsible for managing the costs and environmental effects of the electricity system.
This report is a guide for those trying to interpret the flood of new findings about the electricity used by cryptocurrencies, with a particular focus on the one that garners the most attention (Bitcoin). It only deals with estimates of total electricity use, and does not address questions about the inner workings of cryptocurrency, whether society should be using cryptocurrency, or the pros and cons of cryptocurrency versus other stores of value or media of exchange.
For a clear summary of how Bitcoin works, see Van Valkenburgh.1 For a useful recent summary of the cryptocurrency market and its structure, see Hileman and Rauchs.2 For a useful analysis of the profitability of Bitcoin mining, see Valfells and Egilsson.3
The first section of this report reviews the history of past attempts to estimate electricity used by computing equipment, so recent developments can be seen in historical context. The second section presents complexities and pitfalls of analyzing cryptocurrency technology. The third section evaluates the most widely cited estimates of Bitcoin electricity use. The fourth section summarizes findings from the review of recent estimates. The fifth section gives best practices for doing such analyses properly, derived from the review of previous analyses in sections three and four. The sixth section identifies the most productive areas for future work. Finally, the last section summarizes the findings and best practices so diligent readers can avoid being misled in the future.
Know Your History (Beware of the Hype)
Information technology (IT) changes at a much more rapid pace than many other technologies.4 Unfortunately, many observers unscientifically extrapolate exponential growth rates to create “clickbait” headlines, such as this one in Newsweek on December 11, 2017:
Bitcoin Mining on Track to Consume All of the World’s Energy by 2020
This isn’t the first time people have misunderstood rates of change of information technology. In 2003, writing in IEEE Spectrum, I cited an anecdote from Andrew Odlyzko, formerly at AT&T, now at the University of Minnesota.5 Odlyzko documented the genesis of a familiar “factoid” from the mid-1990s (“the Internet doubles every 100 days”) that led to a substantial overinvestment in the fiber optic network:
[This misconception] … was based on data reported by UUNet, the first commercial Internet service provider, in the mid-1990s. In those days, at least for a brief time, such growth rates actually prevailed. But for almost all the rest of the 1990s, data flows were doubling about every year, as documented by Kerry Coffman and Andrew Odlyzko of AT&T Corp.6 and reported by The Wall Street Journal in 2002.
The difference between the two growth rates is huge: because of the magic of compounding, a doubling of traffic every 100 days translates into growth of about 1000 percent a year, rather than the 100 percent-a-year growth represented by an annual doubling.
During the dot-com boom, industry leaders, investment analysts, and the popular press repeated that gross overestimate of the growth of Internet data flow. The bogus figures helped justify dubious investments in network infrastructure and contributed greatly to overcapacity in the telecommunications and networking industries. Only a tiny fraction of this network capacity is now [circa 2003] being used, and billions of dollars [were] squandered because of blind acceptance of flawed conventional wisdom.
Erroneous reports about information technology’s electricity use emerged in studies around the year 2000,7 at the time of the first dot-com bubble and the California electricity crisis. They popped up again in 20058 and 20139 from the same authors. The claims were reported in major newspapers, cited by investment banking reports and politicians of both political parties, and avidly promoted by people and companies who should have known better.
Those authors claimed that the Internet used 8% of U.S. electricity in 2000, that all computers (including the Internet) used 13%, and that the total would grow to 50% of U.S. electricity in 10 years. The authors also claimed that a wireless Palm VII used as much electricity as a refrigerator (later updated to one iPhone using as much electricity as two refrigerators10) for the networking to support its data flows.
All of these claims turned out to be incorrect,11 but it took years of peer-reviewed research to prove it. We found that the Internet (defined as those authors defined it) used only 1% of U.S. electricity in 2000, all computers together used 3%, the total would never grow to half of all electricity use, and the claim about the wireless Palm VII overestimated networking electricity by a factor of 2,000. In virtually every case, we found that those authors had overestimated electricity used by computing equipment. For a summary of these claims and a review of their effects on investors, see the Epilogue to the 3rd Edition of Turning Numbers into Knowledge.12
Complexities and Pitfalls
The example above came to mind when I first heard about recent claims about Bitcoin electricity use, but the Bitcoin issue has some unique features. Below are some of the factors making assessment of the electricity used by cryptocurrency especially difficult.
Rapid Change
Bitcoin and other cryptocurrencies exhibit rapid rates of change, even faster than growth in normal IT. This rapidity is driven in part by the volatile nature of cryptocurrency prices. Consider the price of Bitcoin, which went up by a factor of about twenty-five during 2017, explaining the substantially increased interest in Bitcoin over that period.13 At this writing (April 12, 2019), Bitcoin prices have fallen to about one-quarter of the price hit at the peak in December 2017. At their nadir in mid-December 2018, they were about one-sixth the peak price.
The price of a cryptocurrency affects profits from mining it, so rapid increases in prices lead to an explosion of new mining facilities coming online. Conversely, drops in prices have dramatic effects in the other direction, inhibiting construction of new facilities and pressuring marginal existing facilities (usually the older ones) to shut down.
The volatility of cryptocurrencies has implications for the electricity system. Consider what would happen to utilities that allocated infrastructure to accommodate Bitcoin mining operations at the peak now that the Bitcoin price has fallen fourfold. If the mining companies go bankrupt, the utility can be stuck with hundreds of thousands of dollars in unpaid power bills as well as the cost of infrastructure upgrades needed to accommodate the installation of those facilities at their inception. Rates of change are far more rapid for cryptocurrency than for utility infrastructure investments, so utility planners need to be careful when planning around cryptocurrency facilities.
Computational Loads and Efficiency
The mathematical calculations required for Bitcoin mining are often performed on servers in data centers. Total electricity use for Bitcoin is affected by the number of servers installed, total computational load, the current mining difficulty of the Bitcoin network, the data centers’ location, and the efficiency of servers and the data centers in which the servers reside. All of these factors can change quickly. Sometimes for information technology, like data centers, growth in efficiency completely offsets growth in computational output,14 but not always (and maybe never in the case of Bitcoin, because of how it is structured). As more computational capacity comes online, the difficulty of the “puzzles” miners need to solve also increases, which may offset in whole or in part the efficiency improvements in computing devices that occur naturally as chips and software improve over time.15
Little to No Tracking of Bitcoin Mining Rigs
Companies like Dataquest and IDC track sales and installed base of conventional servers, but as the Bitcoin mining market has shifted from standard servers to more customized computing devices, it has become more difficult to track the number of servers installed. This creates problems for researchers trying to estimate total electricity used by Bitcoin mining, because the number of servers actively mining is a key factor affecting total electricity use. One key reference is Taylor,16 which makes a preliminary assessment of this equipment’s characteristics.
Few Measured Data on Energy Use and Efficiency
There are few measured energy data on the electricity use and efficiency of Bitcoin mining servers and the facilities in which they are installed. Researchers have learned through experience that manufacturers’ estimates of power use cannot always be taken at face value. The only way to create replicable results is to simultaneously measure power use and computing output using standardized, accurate, reliable, reproducible, and representative protocols.17
Simple assumptions about the power use of one kind of equipment for Bitcoin mining can lead to problems. In the first credible analysis of its kind, O’Dwyer and Malone included a table of the power drawn for Bitcoin mining using different kinds of computing equipment.18 They relied on manufacturer-reported data from Amazon.com listings for seven different kinds of computers (and one other manufacturer source for an eighth), ranging from general purpose computers to more recent Application Specific Integrated Circuits (ASICs).
The efficiency of computing devices often improves over time, as hardware and software get better. The table in the 2014 O’Dwyer and Malone study lists PlayStation 3 and gives a power estimate of 60 watts when mining for Bitcoin, but doesn’t specify the year in which that console chassis was released. Earlier versions of the PlayStation 3 used closer to 180 watts at full output,19 so imprecision in specifying device types, firmware versions, and vintages can lead to confusion and inaccuracy.
The more general problem is that the computer scientists who have conducted laudable and pathbreaking early work in assessing Bitcoin electricity use are not always familiar with the literature, procedures, and pitfalls associated with measuring the electricity use of computing equipment. For example, the O’Dwyer and Malone study didn’t include the power used by supporting infrastructure and equipment (e.g., cooling, fans, pumps, and power distribution losses). Fortunately, the effect of this omission was limited, at least in part, because Bitcoin mining still wasn’t quite as big an industrial enterprise back then, and many mining rigs were running without such infrastructure at that point.
Secrecy of Bitcoin Supply Chain
Bitcoin miners don’t share many details about their mining facilities, hardware, and software. The rapid growth in Bitcoin also accentuates the tendency towards secrecy. Mining companies are of comparatively recent origin, and they’ve often experienced explosive growth, which can mean that they haven’t yet allocated staff resources to tracking and reporting data, and don’t have solid systems in place as more established companies might.
Brandolini’s Law Governs
In 2014, an Italian software developer named Alberto Brandolini made the following trenchant observation, which has come to be known as Brandolini’s law, or the nonsense asymmetry principle:
The amount of energy needed to refute [nonsense] is an order of magnitude bigger than to produce it.20
This “law” encapsulates one important reality of living in the digital age: it is easier to create ostensibly accurate but incorrect claims than it is to demonstrate why they are invalid. That is the nature of topics requiring careful analysis, but people have come to expect instant answers on new topics, even when there are no real data and research. That’s the case for Bitcoin electricity use, which has emerged as a topic of wide public interest only in the past few years.
My corollary to Brandolini’s law is that
In fast-changing fields, like information technology, refutations lag nonsense production to a greater degree than in fields with less rapid change.
This corollary places special obligations on media producers and consumers21 to use care in interpreting and citing ostensibly credible statistics.
Evaluating Existing Independent Estimates of Bitcoin Electricity Use
There have been surprisingly few original estimates of Bitcoin electricity use, and almost all the estimates appearing in the media before late 2018 relied heavily or exclusively on the estimates from the website Digiconomist. I review the key estimates from the academic literature below, and have largely excluded reports and white papers in favor of refereed journal articles (or, in the case of Bevand, detailed commentary on the analysis of Digiconomist, supported by well-documented calculations). In the next section, I present a summary assessment of these independent estimates.
2014 O’Dwyer and Malone
To my knowledge, O’Dwyer and Malone22 were the first academic analysts to publish estimates of total Bitcoin electricity use. Their conference paper, which takes a top-down view of Bitcoin mining and associated electricity use, estimates both the total computational output devoted to Bitcoin (using characteristics of the algorithm for Bitcoin mining) and the efficiency of different equipment in computational output per watt. Dividing total computational output by efficiency yields the total watts devoted to mining Bitcoin at any time, which the authors estimate to range between 0.01 GW23 and 10 GW as of March 2014.24
The given range is mainly a function of the difference in efficiency between the most and least efficient hardware systems that their article reviewed. O’Dwyer and Malone also state that a number of 3 GW (about the average power use of Ireland) is a plausible best estimate, but don’t explain how they drew this conclusion from their stated range. It is unclear why choosing a number that is half an order of magnitude from the top end of the range makes sense when the total range is three orders of magnitude.
There are several issues with this way of estimating Bitcoin power use. The authors don’t explore the possibility that the economics of Bitcoin mining might bias the actual stock of equipment in use towards the more efficient devices, thus making the total more likely to reside closer to the lower end of their range. While the authors deserve credit for stating a range, the lack of real stock efficiency data makes it hard to interpret what that range really means. Additionally, their estimates ignore the infrastructure overhead electricity use (e.g., fans, cooling, pumps, power distribution losses), which vary between 5% and 20% of computing electricity use in most new conventional data centers.
Fortunately, the authors don’t represent their calculations as anything more than an initial rough estimate, and they did a credible job of creating their estimates using simple data. The estimates depend on a few parameters, with the greatest uncertainty arising from the efficiency of mining equipment currently in use. The authors avoid doing projections, thankfully, and don’t address greenhouse gas emissions at all.
The media, though, were not so careful when citing this study. A careful reading of this admittedly early-stage research suggests that the 3 GW number is too speculative and preliminary to rely upon.
2016–2018 Digiconomist
The website Digiconomist25 is the source for many media estimates of Bitcoin electricity use.26 The site is the creation of Alex de Vries, an economist in the Netherlands, and he summarizes his estimates in the form of a “Bitcoin energy consumption index.”27 Figure 1 is a graph of that index for roughly 27 months from February 2017 to mid-April 2019, showing TWh (terawatt-hours or billion kWh) on the Y-axis and time on the X-axis.
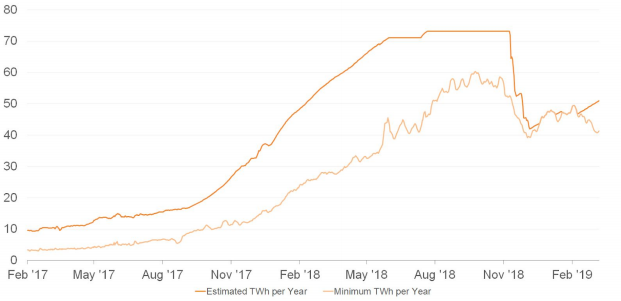
De Vries published a commentary article in the peer-reviewed journal Joule, summarizing the method for generating the Bitcoin energy consumption index.28 De Vries is able to create a time series day-by-day because he generates the index using the following data:
1) the average price of Bitcoin over some time period (it was about $5,000 as of this writing, April 12, 2019);
2) the number of Bitcoins mined over that same time period;
3) an assumption about the percentage of Bitcoin mining revenues that is spent on electricity (60%), which would include both direct electricity use for Bitcoin mining rigs and the supporting infrastructure electricity (cooling, fans, pumps, power conversion and distribution, etc); and
4) an assumption about the average price of electricity (5¢ U.S./kWh) for Bitcoin miners.
The average price of Bitcoin over some time period times the number of Bitcoins mined in that period plus transaction fees gives total revenues, which are multiplied by 60% to get electricity expenditures. Those expenditures are then divided by the assumed electricity price to get estimated electricity consumption of the network for the analysis period.
This calculation has the advantage of being tractable using available data, but it relies on assumptions about economic parameters and Bitcoin miner behavior (e.g., optimal allocation of mining resources) to estimate physical characteristics of a technological system.
Such assumption-based calculations are prone to error. Economic parameters (like Bitcoin prices) are volatile, and are at best imperfectly correlated with electricity use. Real economic systems may tend toward optimality, but they often don’t get close because of transaction costs, cognitive failures, and other imperfections in people and institutions. In addition, the assumptions used by Digiconomist (like 60% of Bitcoin revenues equalling electricity costs and 5¢ U.S./kWh being the electricity price) are so simplistic as to make any careful analyst wary.
Given Digiconomist’s stated methodology, it is unclear why Bitcoin electricity consumption should be flat (at exactly 73.12146 TWh/year) from July 31, 2018 through November 19, 2018 when the Bitcoin price during this period varied by more than 40%. Finally, Digiconomist doesn’t reveal the periods over which its methodology averages the price of Bitcoin, and these periods have apparently changed over time, according to Bevand (see below). This lack of transparency must be rectified before anyone should consider these data an accurate depiction of Bitcoin’s electricity use.
The more direct way to estimate electricity used by Bitcoin mining equipment is to understand the characteristics of the underlying computing, cooling, and power distribution equipment using field data (as my co-authors and I did for our most recent data center report).29 Unfortunately, aside from a few anecdotes in the trade and popular press, there’s little information available on Bitcoin mining operations.
The servers used for Bitcoin mining are nowadays customized for just that application. They bear little resemblance to standard server hardware installed in corporate or hyperscale data centers. Because these custom servers have not until recently been tracked by the big data providers (e.g., IDC and Gartner), we have only guesses and estimates of how many are being built and run. We know almost nothing about where they’re being installed. And our knowledge of the power systems and cooling equipment in these facilities is virtually nil.
These data issues make it hard to create accurate estimates of electricity used by Bitcoin mining in the aggregate. This is probably why De Vries used his simplified economic approach to generate his index, but that doesn’t mean this method is a reliable indicator of Bitcoin electricity use, or probably even its trends over time.
2017 and 2018 Bevand
Marc Bevand, an “analyst and crypto[currency] entrepreneur,”30 assessed the Digiconomist estimates on February 1, 2017.31 Most importantly, he expressed concern over the arbitrary assumption of 60% of revenues paying for electricity, and showed a range in his data (based on real equipment) of between 6.3% and 38.6%—implying far lower electricity use if his data were indeed representative.32 Using a more refined approach and more detailed information about the characteristics of Bitcoin mining rigs over time, he estimated Bitcoin electricity use for February 26, 2017, July 28, 2017, and January 11, 2018.
Bevand did not generate day-by-day estimates for the intervening periods as Digiconomist does, but these three data points are enough to teach some important lessons, as we discuss below. Both sources show growth of about fourfold between February 26, 2017 and January 11, 2018. That’s rapid growth compared to conventional end-uses, so it’s not surprising that Bitcoin is getting more attention. The big difference between Bevand and Digiconomist is in the absolute electricity consumption. Bevand’s estimates are less than half of the Digiconomist estimates. They also are far more technically detailed, less dependent on high-level arbitrary assumptions, and presented with reasonable high-low error bars. In my view, these attributes make Bevand’s estimates more trustworthy.
October 2017 Vranken Article in Current Opinion in Environmental Sustainability
One of the more carefully crafted estimates of Bitcoin electricity use was published by Vranken in late 2017, before the huge run-up of Bitcoin prices in 2018.33 It uses a similar method to Bevand and O’Dwyer and Malone, but with updated data, and focuses on estimating a range of consumption for January 1, 2017.
This article is exemplary in that it explicitly considers the economics of Bitcoin mining rigs in the face of technical progress, electricity prices, and Bitcoin prices. The economics of mining provide critically important constraints on which kinds of mining rigs can be economical under different conditions, and the best analyses in the future will use such models to hone their estimates of electricity use. The article concludes that a range of 0.1 to 0.5 GW is a reasonable estimate for Bitcoin electricity use as of January 1, 2017.
October 2018 Mora et al. Article in Nature Climate Change
One of the most dramatic recent estimates of Bitcoin electricity use, focusing on current and projected CO2 emissions from that electricity consumption, was published by Mora et al. in the peer-reviewed journal Nature Climate Change.34 This study is problematic in many ways, not least for its sensational title, its extrapolation over many decades, and its limited documentation of data and methods.35 The title of the article is “Bitcoin emissions alone could push global warming above 2°C.” Given the flaws in the analysis, however, that conclusion is also almost certainly wrong.
The article and the supplemental material do not report electricity use of Bitcoin at all, just emissions estimates, but it is possible to reproduce the authors’ calculations and estimate electricity use for the year 2017 (the article only gives the year to which its estimates apply).36 The authors assumed that the efficiency of Bitcoin mining as well as the emissions intensity of electricity generation would stay constant over the next century. They also chose a method that led to a substantial overestimate of base-year (2017) electricity used by Bitcoin. For more details, see the rebuttal to the Mora et al. article we submitted in April 2019.37
November 2018 Krause and Tolaymat Article in Nature Sustainability
Krause and Tolaymat published an article in the peer-reviewed journal Nature Sustainability in late 2018 that contained exemplary documentation and credible analysis, with some issues.38 Their data, laid out clearly in the article’s supplemental materials, cover the period from January 1, 2016 through June 30, 2018, and the article’s stated purpose is to compare energy and resource intensities for four cryptocurrencies with more conventional currencies.
This comparison is complicated for many reasons, in part because cryptocurrency can be both a store of value and a medium of exchange, and different conventional currencies serve either or both of these purposes to different degrees in different societies and at different times. The article attempts to compare energy consumption between different currency options, but does not document how the authors convert electricity to primary energy39 (which has the potential to introduce a factor-of-three error in energy consumption calculations), giving little confidence that they have correctly treated this particular issue for purposes of their comparison. The article also presents annual results and averages some results over a period of more than two years—inappropriate for Bitcoin, as things change too fast for such long averages to be meaningful.
Those issues do not affect the data on electricity used by Bitcoin, which are reported day-by-day for the period spanning January 1, 2016 through June 30, 2018 in the supplemental materials. It is straightforward to assess their data and methods through those materials, which gives me confidence that they did a relatively credible analysis on electricity used by Bitcoin mining. They found numbers similar in size to those of Bevand and Vranken, which are the two other analyses of highest credibility assessed here.
Summary of Independent Estimates
Table 1 summarizes various characteristics of the estimates analyzed in this report. The most important conclusion from this review is that proper estimates of Bitcoin electricity use require far more actual measured data, which will allow detailed bottom-up estimates that are currently impossible. Right now all of these estimates treat uncertainty incompletely, if at all, and don’t deal with the large geographic variations in electricity prices and emissions per kWh that dominate both the economic analysis and emissions calculations for Bitcoin electricity use. None of them appear to include infrastructure overhead electricity use, which might add 5–20% to these totals (with the caveat that nobody has published measurements of infrastructure overhead for these facilities).
Figure 2 summarizes Bitcoin electricity use estimates for six different studies over time. The three most credible estimates (Bevand, Vranken, and Krause and Tolaymat) are comparable, while the other three estimates are outliers in various ways. The O’Dwyer and Malone estimate gives a massive range (which is understandable because it was the first attempt at such analysis), but inexplicably chooses a “midpoint” number that is nowhere near the midpoint between its high and low estimates (and is biased towards the top end). Digiconomist shows numbers that are consistently about a factor of two higher than the more credible estimates, while the Mora et al. analysis has implied electricity use that is 5 to 40 times bigger than that of the more credible estimates in 2017.
The world used about 22,000 TWh in 2017,40 the latest year for which I could find historical data, and this number historically increases by only a few percent a year. This means that on January 11, 2018, Bitcoin accounted for about 0.1% of global electricity if Bevand’s numbers are correct. Krause and Tolaymat estimated power use comparable to Bevand’s numbers for January 11, 2018, and further estimated power use to grow to be about twice as much by June 30, 2018, so that places Bitcoin electricity use at about 0.2% of global electricity use by mid-2018.41 For comparison, all conventional data centers (which don’t include Bitcoin electricity use) consumed about 200 TWh in 2018,42 so Bitcoin adds about 20–25% to conventional data center consumption.
Interestingly, Krause and Tolaymat’s estimates (as well as the less reliable ones from Digiconomist) show Bitcoin electricity use starting to decline in mid-2018 following the collapse in Bitcoin prices. Only time will tell if this trend will continue.
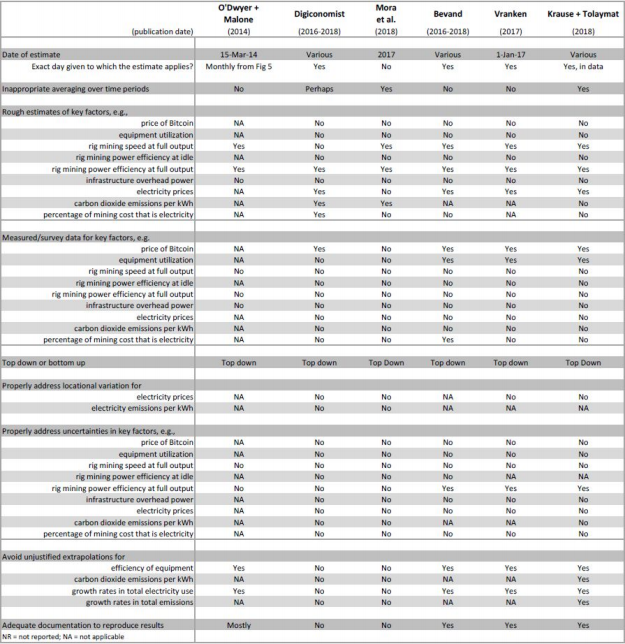
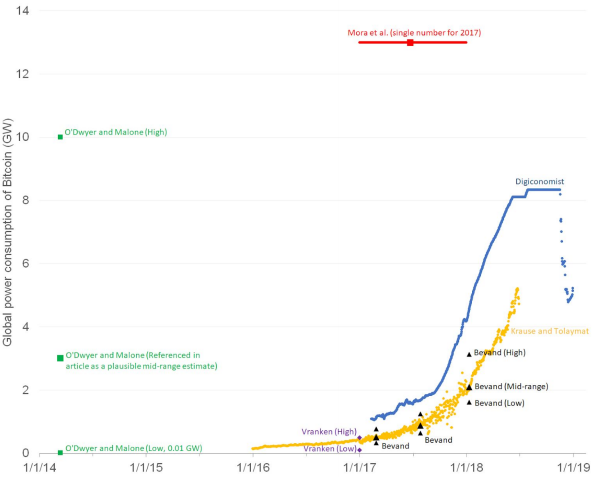
Doing It Right
Here are my proposed best practices for doing accurate analyses in this space, based on what I learned by assessing previous efforts.
Report Estimates to the Day
One important feature of cryptocurrency electricity use is the need to specify the exact day to which an estimate applies (some methods allow for day-by-day tracking). That’s because Bitcoin mining is changing rapidly over time, so generating a monthly or annual average isn’t accurate enough. Such rapid change also makes estimates from only a couple of years back less useful than one might expect for estimating present-day Bitcoin electricity use.
As a corollary, values over significant time periods shouldn’t be averaged, except in rare circumstances. Many analytical studies do such averaging because, for normal systems, it’s often OK. For rapidly changing things like cryptocurrency, however, it’s a recipe for disaster. Of course, it’s important to choose a date of analysis to accurately portray recent trends—if there have been rapid recent changes in Bitcoin price, for example, it’s important to show how that might affect your results.
Provide Complete and Accurate Documentation
As a general rule, analyses that don’t provide enough documentation for any competent researcher to reproduce the results are not to be trusted. As I describe in the 3rd edition of my book, Turning Numbers into Knowledge, documentation gives credit where credit is due, leaves a trail so you can remember, leaves a trail for others to follow, and helps you check your analysis.43 Some of the analyses described above provided superb documentation, while others fell short.
Avoid Guesses and Rough Estimates About Underlying Data
It is common practice when data aren’t available to make assumptions for key parameters, based on intuition, common knowledge, or physical principles. The problem for new and fast-changing systems like cryptocurrencies is that such assumptions are unlikely to be correct. I’m often reminded of the prescient quotation addressing long-term projections of energy systems from the economist E. F. Schumacher, but he could as well have been talking about the topic of this report:
It is fashionable today to assume that any figures about the future are better than none. To produce figures about the unknown, the current method is to make a guess about something or other — called an “assumption” — and to derive an estimate from it by subtle calculation. The estimate is then presented as the result of scientific reasoning, something far superior to mere guesswork. This is a pernicious practice that can only lead to the most colossal planning errors, because it offers a bogus answer where, in fact, an entrepreneurial judgment is required.44
Because of the lack of data about these systems, assumptions are rampant in estimates of electricity use for Bitcoin and other cryptocurrencies. Where key parameters are unknown, it’s better to collect data instead of making assumptions, and if data aren’t available, it’s better not to publish estimates at all, or to couch the results in an appropriately cautious way, recognizing the limitations of assumptions in this fast-moving space.
Collect Measured Data in the Field for Both Components and Systems
The importance of measured data for understanding a new area of human activity can’t be overestimated. We need real-time measurements of power used by Bitcoin servers while mining, so power can be correlated with performance. And we don’t just need annual averages or power at maximum output; we need to know how the servers perform when not fully loaded.
Many in the industry assume that Bitcoin mining servers will be operating at maximum output most of the time, but there is no public dataset of measured real-time utilization levels for this equipment. We need measurements of utilization to understand the conditions under which Bitcoin rigs might be less than 100% utilized.
There are few data on the operational characteristics of the facilities in which Bitcoin mining rigs are housed, but these are likely to be different than conventional data centers (for which some measured field data are available). We need measurements of the overhead associated with power systems, fans, pumps and cooling, so we can compare those to the characteristics of more traditional facilities.45 One potential difference is that some Bitcoin mining rigs use liquid cooling to the chip—uncommon in traditional data centers—while others use only ambient air for cooling. How common are these practices for Bitcoin mining? Nobody knows without field data and measurements.
Build from the Bottom Up
The most accurate estimates of electricity used by data centers come from detailed data built up from the number of computing systems in the field, combined with measurements of their efficiency (performance per watt) and other operational characteristics, and aggregated to total electricity use.46 The alternative is making high-level assumptions (guesses) and rough calculations that are prone to error for systems for which good data don’t yet exist. Any estimates that aren’t built from the bottom up using measured data are suspect, especially in the early days of rapidly evolving new technologies used in highly volatile markets.
All of the estimates reviewed above rely to some extent on top-down assumptions about efficiency of equipment, because measured data and information about the stock and characteristics of Bitcoin mining rigs just don’t exist for the industry as a whole.
Properly Address Locational Variations in Siting of Mining Facilities
The price of electricity is a key variable for understanding where Bitcoin mining facilities are located, because electricity cost affects profitability of these rigs. For the U.S., where data are widely available, industrial electricity prices varied by a factor of 5 from the lowest-priced state to the highest-priced state in 201847 (and probably more if intra-state variations are tallied). Similar price variations exist across the globe. Such variation implies that Bitcoin miners, who are notoriously price sensitive, are much more likely to seek out those low-priced areas, such as those where cheap hydroelectric power dominates.
The incentive to cluster where electricity prices are cheap also affects estimates of emissions from Bitcoin mining. Average emissions factors for electricity systems vary even more than prices. Hydroelectric dams don’t emit CO2, although their output may otherwise be used to displace CO2-emitting fossil-fueled generation elsewhere, depending on local transmission, market, and hydrological conditions.
These variations mean that it is not sufficient to use national electricity prices and emissions factors to estimate mining costs and emissions, and analyses that rely on national average factors are likely to be misleading or wrong. To analyze the economics and emissions from Bitcoin mining, best practice would therefore be to identify exact locations of mining facilities.
Explicitly and Completely Assess Uncertainties
In any new area of research, there are significant uncertainties. We don’t know enough about the characteristics of Bitcoin mining rigs and the facilities in which they’re housed, or about how and where those facilities are operated. We know little about the economics of Bitcoin mining and the incentives to expand or contract mining operations. All of these uncertainties should give pause to anyone estimating the electricity used by Bitcoin or other cryptocurrencies. When making such estimates, it’s important, as much as possible, to characterize the full range of uncertainty associated with key drivers of that electricity use. Where uncertainties can’t be estimated, researchers should present those uncertainties qualitatively, discuss their potential importance, and use due caution in presenting their results.
Avoid Extrapolating into the Future
The physicist Niels Bohr once famously said “Predictions are difficult, especially about the future.” Some of the most egregious missteps in recent estimates of Bitcoin electricity use have occurred when observers make wild extrapolations into the future using limited data. Because information technology changes so fast, it is always possible to find rapid growth rates that when extrapolated for a year or two will lead to “gee whiz!” projections that will shock your readers. Doing this creates misunderstandings that may take years to correct, and may in the meantime cause misinformed people to waste precious resources, time, and opportunities.
Even well-founded projections for information technology should be limited to a few years ahead, because things in this industry change so fast. Nobody should make projections of growth in IT electricity use for one or two decades hence. And nobody should assume that important parameters like device efficiency or emissions intensity per kWh will remain constant for long periods of time.
Future Work
Surveys and Field Measurements
The most critical research need is for surveys of equipment characteristics and field measurements of equipment affecting Bitcoin electricity use. We know little about how Bitcoin mining is actually done in real facilities, and until more field data are collected, it will be difficult to make estimates of total Bitcoin electricity use more accurate. We’ll also need to carefully assess changes in technology over time.
The Economics of Bitcoin Mining
Creating a credible model of the economics of Bitcoin mining as a function of electricity prices, Bitcoin prices, and other variables is important for predicting the future of electricity consumption for mining. Because Bitcoin prices are so volatile, the economics are a strong driver of whether the amount of electricity used to mine Bitcoin will increase or decrease over time. The estimates that included economic assessments of mining generally did better in estimating which mining rigs were likely in place at any particular time, and so were more accurate in their assessments of total electricity use.
Examining Electricity Intensity of Other Cryptocurrencies
Bitcoin is only one of many ways to structure a cryptocurrency. Careful comparison among other options may yield insights as to how to accomplish the same goals at vastly lower electricity and emissions intensity. Future studies should examine competing models for using blockchains to implement cryptocurrencies.
Conclusions
The lure of clickbait headlines is antithetical to good research. As in the past for other areas of information technology, some people’s desire for catchy conclusions has led them to make consequential errors in analyzing Bitcoin electricity use (almost always overestimating this use).
I’ve identified three credible estimates of Bitcoin electricity use that agree with each other well—Bevand, Vranken, and Krause and Tolaymat—and three others that have serious issues—O’Dwyer and Malone, Digiconomist, and Mora et al. The latest estimate from one of these credible sources is about 5 GW of power use globally on June 30, 2018, with some indications that electricity use started falling around that time, due to the collapse of Bitcoin prices in early 2018. If converted to annual electricity consumption, 5 GW represents 44 billion kWh (44 TWh), or about 0.2% of global electricity use.
Notes
- Peter Van Valkenburgh, “What is Bitcoin Mining and Why is it Necessary?” Coin Center (Dec. 15, 2014) https://coincenter.org/entry/what-is-bitcoin-mining-and-why-is-it-necessary. ↩
- Garrick Hileman and Michel Rauchs, “Global Cryptocurrency Benchmarking Study,” University of Cambridge Centre for Alternative Finance (Apr. 6, 2017) https://dx.doi.org/10.2139/ssrn.2965436. ↩
- Sveinn Valfells and Jón Helgi Egilsson, “Minting Money With Megawatts,” Proceedings of the IEEE, Vol. 104, No. 9 (Sep. 2016): pgs. 1674-1678, https://ieeexplore.ieee.org/document/7547426. ↩
- William D. Nordhaus, “Two Centuries of Productivity Growth in Computing,” Journal of Economic History, Vol. 67, No. 1 (Mar. 2007): pgs. 128-159, https://doi.org/10.1017/S0022050707000058; Jonathan Koomey and Samuel Naffziger, “Energy efficiency of computing: What’s next?” Electronic Design (Nov. 28, 2016) https://www.electronicdesign.com/microprocessors/energy-efficiency-computing-what-s-next; Jonathan G. Koomey, H. Scott Matthews, and Eric Williams, “Smart Everything: Will Intelligent Systems Reduce Resource Use?,” Annual Review of Environment and Resources, Vol. 38 (Oct. 2013): pgs. 311-343, https://doi.org/10.1146/annurev-environ-021512-110549; Jonathan G. Koomey, Stephen Berard, Marla Sanchez, and Henry Wong, “Implications of Historical Trends in The Electrical Efficiency of Computing,” IEEE Annals of the History of Computing, Vol. 33, No. 3 (Jul.–Sep., 2011): pgs. 46-54, https://doi.ieeecomputersociety.org/10.1109/MAHC.2010.28. ↩
- J. G. Koomey, “Sorry, wrong number – How to separate fact from fiction in the information age,” IEEE Spectrum, Vol. 40, No. 6 (Jun. 2003): pgs. 11-12, https://ieeexplore.ieee.org/document/1203076. ↩
- K. G. Coffman and A. M. Odlyzko, “Growth of the Internet,” AT&T Labs Research White Paper (Jul. 6, 2001) http://www.dtc.umn.edu/~odlyzko/doc/oft.internet.growth.pdf. ↩
- Jonathan Koomey, “Epilogue.” In Turning Numbers into Knowledge: Mastering the Art of Problem Solving. 3rd ed. El Dorado Hills, CA: Analytics Press (2017). ↩
- Ibid, 219. ↩
- Jon Koomey, “Does Your iPhone Use As Much Electricity As A New Refrigerator? Not Even Close.” ThinkProgress (Aug. 25, 2013) https://thinkprogress.org/does-your-iphone-use-as-much-electricity-as-a-new-refrigerator-not-even-close-4a5e0ab41a13. ↩
- Id. ↩
- Jonathan G. Koomey, “Wild claims about electricity used by computers that just won’t die (but should),” Blog (Aug. 19, 2013) https://www.koomey.com/post/58707377661. ↩
- Jonathan G. Koomey, “Epilogue.” In Turning Numbers into Knowledge: Mastering the Art of Problem Solving, El Dorado Hills, CA: Analytics Press, (2017): 213-222. ↩
- Numbers reported by http://www.bitcoinity.org. ↩
- Arman Shehabi, Sarah J. Smith, Eric Masanet, and Jonathan Koomey, “Data Center Growth in the United States: Decoupling the Demand for Services from Electricity Use,” Environmental Research Letters, Vol. 13, No. 12 (Dec. 18, 2018): pgs. 1-11, https://dx.doi.org/10.1088/1748-9326/aaec9c. ↩
- Jonathan Koomey and Samuel Naffziger, “Energy efficiency of computing: What’s next?” Electronic Design (Nov. 28, 2016) https://www.electronicdesign.com/microprocessors/energy-efficiency-computing-what-s-next. ↩
- Michael Bedford Taylor, “The Evolution of Bitcoin Hardware,” Computer, Vol. 50 (Sep. 2017): pgs. 58-66, https://doi.ieeecomputersociety.org/10.1109/MC.2017.3571056. ↩
- Jonathan Koomey, et al., Server Energy Management Protocol, Oakland, CA: Analytics Press (Nov. 3, 2006) available at: https://www.energystar.gov/ia/products/downloads/Finalserverenergyprotocol-v1.pdf. ↩
- Karl J. O’Dwyer and David Malone, “Bitcoin Mining and its Energy Footprint,” 25th IET Irish Signals & Systems Conference 2014 and 2014 China-Ireland International Conference on Information and Communications Technologies (ISSC 2014/CIICT 2014) (Sep. 29, 2014) https://doi.org/10.1049/cp.2014.0699. ↩
- Amanda Webb, Kieren Mayers, Chris France, and Jonathan Koomey, “Estimating the Energy Use of High-Definition Games Consoles,” Energy Policy, Vol. 61 (Oct. 2013): pgs. 1412-1421, https://doi.org/10.1016/j.enpol.2013.05.056. ↩
- Guillaume Nicoulaud, “Brandolini’s law,” Ordre Spontané Blog (Jul. 8, 2014) https://ordrespontane.blogspot.com/2014/07/brandolinis-law.html. ↩
- Jonathan Koomey, “Separating Fact from Fiction: A Challenge for the Media,” IEEE Consumer Electronics Magazine, Vol. 3, No. 1 (Jan. 2014): pgs. 9-11, https://doi.org/10.1109/MCE.2013.2284952. ↩
- Karl J. O’Dwyer and David Malone, “Bitcoin Mining and its Energy Footprint,” 25th IET Irish Signals & Systems Conference 2014 and 2014 China-Ireland International Conference on Information and Communications Technologies (ISSC 2014/CIICT 2014) (Sep. 29, 2014) https://doi.org/10.1049/cp.2014.0699. ↩
- The authors state that the low end of the range is 0.1 GW (100 MW) but Figure 5 indicates that it’s actually 0.01 GW (10 MW). That makes sense because the efficiency range used to derive the total power use in their study is three orders of magnitude, so the total electricity use should also range by three orders of magnitude. ↩
- Their Figure 5 gives estimates over time from January 2009 to March 2014. ↩
- Available at https://digiconomist.net. ↩
- Jonathan G. Koomey, “Updated list of articles on Bitcoin electricity use and related topics,” Blog (Mar. 12, 2018) https://www.koomey.com/post/171808646518. ↩
- See: “Bitcoin Energy Consumption Index,” Digiconomist, accessed Apr. 23, 2019, https://digiconomist.net/bitcoin-energy-consumption. ↩
- Alex de Vries, “Bitcoin’s Growing Energy Problem,” Joule, Vol. 2, No. 5 (May 16, 2018): pgs. 801-805, https://doi.org/10.1016/j.joule.2018.04.016. ↩
- Arman Shehabi, et al., “United States Data Center Energy Usage Report,” Ernest Orlando Lawrence Berkeley National Laboratory Report, LBML-1005775 (Jun. 2016) http://eta-publications.lbl.gov/sites/default/files/lbnl-1005775_v2.pdf. ↩
- Robert Walton, “As concern grows over bitcoin’s energy use, what’s next for cryptocurrency?,” Utility Dive (Mar. 28, 2018) https://www.utilitydive.com/news/as-concern-grows-over-bitcoins-energy-use-whats-next-for-cryptocurrency/519859. ↩
- Marc Bevand, “Serious faults in Digiconomist’s Bitcoin Energy Consumption Index,” Blog (Feb. 1, 2017) http://blog.zorinaq.com/serious-faults-in-beci. ↩
- Marc Bevand, “Electricity consumption of Bitcoin: a market-based and technical analysis,” Blog (Mar. 10, 2017) http://blog.zorinaq.com/bitcoin-electricity-consumption. ↩
- Harald Vranken, “Sustainability of bitcoin and blockchains,” Current Opinion in Environmental Sustainability, Vol. 28 (Oct. 2017): pgs. 1-9, https://doi.org/10.1016/j.cosust.2017.04.011. ↩
- Camilo Mora, et al., “Bitcoin emissions alone could push global warming above 2°C,” Nature Climate Change, Vol. 8 (Oct. 19, 2018): pgs. 931-933, https://doi.org/10.1038/s41558-018-0321-8. ↩
- Eric Masanet, Arman Shehabi, Nuoa Lei, Harald Vranken, Jonathan Koomey, and Jens Malmodin, “Implausible projections overestimate near-term Bitcoin CO2 emissions,” Submitted to Nature Climate Change (Mar. 3, 2019). ↩
- I am indebted to Professor Eric Masanet of Northwestern University for extracting the implied electricity consumption figures from the Mora et al. analysis. ↩
- Eric Masanet, Arman Shehabi, Nuoa Lei, Harald Vranken, Jonathan Koomey, and Jens Malmodin, “Implausible projections overestimate near-term Bitcoin CO2 emissions,” Submitted to Nature Climate Change (Mar. 3, 2019). ↩
- Max J. Krause and Thabet Tolaymat, “Quantification of energy and carbon costs for mining cryptocurrencies,” Nature Sustainability, Vol. 1, No. 11 (Nov. 1, 2018): pgs. 711-718, https://doi.org/10.1038/s41893-018-0152-7. ↩
- Jonathan Koomey, Zachary Schmidt, Holmes Hummel, and John Weyant, “Inside the Black Box: Understanding key drivers of global emission scenarios,” Environmental Modelling & Software, Vol. 111 (Jan. 2019): pgs. 268-281, https://doi.org/10.1016/j.envsoft.2018.08.019. ↩
- This total is sales of electricity plus direct (on-site) generation. See: https://yearbook.enerdata.net/electricity/electricity-domestic-consumption-data.html. Sometimes people report total electricity generation, which is typically higher than consumption for the electricity grid by 6-8% in developed countries (because of line losses to transport electricity to end users). ↩
- Max J. Krause and Thabet Tolaymat, “Quantification of energy and carbon costs for mining cryptocurrencies,” Nature Sustainability, Vol. 1, No. 11 (Nov. 1, 2018): pgs. 711-718, https://doi.org/10.1038/s41893-018-0152-7. ↩
- Dave Turk and Laura Cozzi, et al., “Digitalization & Energy,” International Energy Agency (Nov. 5, 2017) https://www.iea.org/digital. ↩
- Jonathan G. Koomey, Turning Numbers into Knowledge: Mastering the Art of Problem Solving, El Dorado Hills, CA: Analytics Press (2017). ↩
- E. F. Schumacher, Small Is Beautiful: A Collection of Essays As If People Mattered, London: Blond & Briggs (1973) pgs. 90-91. ↩
- Arman Shehabi, et al., “United States Data Center Energy Usage Report,” Berkeley, CA: Lawrence Berkeley National Laboratory. LBNL-1005775, (Jun. 2016), https://eta.lbl.gov/publications/united-states-data-center-energy. ↩
- Ibid. ↩
- U.S. Department of Energy, “Electric Power Monthly (for December 2018),” Washington, DC: U.S. Energy Information Administration, accessed Feb. 27, 2019, https://www.eia.gov/electricity/monthly/archive/december2018.pdf. ↩